Causal Machine Learning
Last updated on
Fri, Nov 5, 2021
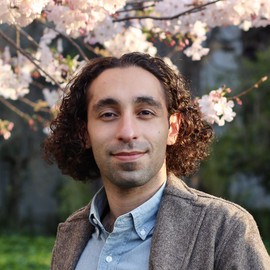
Nima Hejazi
Assistant Professor of Biostatistics
My research lies at the intersection of causal inference and machine learning, developing flexible methodology for statistical inference tailored to modern experiments and observational studies in the biomedical and public health sciences.
Related
Publications
A generalization of moderated statistics to data adaptive semiparametric estimation in high-dimensional biology
The widespread availability of high-dimensional biological data has made the simultaneous screening of many biological characteristics …
Doubly-robust inference in R using drtmle
Inverse probability of treatment weighted estimators and doubly robust estimators (including augmented inverse probability of treatment …
David Benkeser, Nima Hejazi
Revisiting the propensity score's central role: Towards bridging balance and efficiency in the era of causal machine learning
About forty years ago, in a now–seminal contribution, Rosenbaum and Rubin (1983) introduced a critical characterization of the …
Nima Hejazi, Mark van der Laan
haldensify: Highly adaptive lasso conditional density estimation in R
The haldensify R package serves as a toolbox for nonparametric conditional density estimation based on the highly adaptive lasso, a …
Efficient estimation of modified treatment policy effects based on the generalized propensity score
Continuous treatments have posed a significant challenge for causal inference, both in the formulation and identification of …
Causal survival analysis under competing risks using longitudinal modified treatment policies
Longitudinal modified treatment policies (LMTP) have been recently developed as a novel method to define and estimate causal parameters …
A framework for causal segmentation analysis with machine learning in large-scale digital experiments
We present an end-to-end methodological framework for causal segment discovery that aims to uncover differential impacts of treatments …
Talks
Combining Causal Inference and Machine Learning for Model-agnostic Discovery in High-dimensional Biology
The widespread availability of high-dimensional data has catalyzed biological pattern discovery. Today, the simultaneous measurement of …
Mon, Mar 27, 2023 1:00 PM
Boston, Massachusetts, USA
Evaluating Treatment Efficacy in Clinical Trials with Two-phase Designs Using Stochastic-interventional Causal Effects
In clinical trials randomizing participants to active vs. control conditions and following units until the occurrence of a primary …
Mon, Dec 19, 2022 4:20 PM
London, England, UK
Using Stochastic-interventional Causal Effects to Evaluate Treatment Efficacy in Clinical Trials
In clinical trials randomizing participants to active vs. control conditions and following study units until the occurrence of a …
Thu, Dec 1, 2022 12:00 PM
Boston, MA, USA
Combining Causal Inference and Machine Learning for Model-agnostic Discovery in High-dimensional Biology
The widespread availability of high-dimensional data has catalyzed the process of biological pattern discovery. Today, the simultaneous …
Fri, Nov 4, 2022 10:00 AM
Edinburgh, Scotland, UK
Evaluating Treatment Efficacy in Vaccine Clinical Trials with Two-phase Designs Using Stochastic-interventional Causal Effects
In clinical trials randomizing participants to active vs. control conditions and following study units until the occurrence of a …
Wed, Oct 5, 2022 12:00 PM
Cambridge, MA, USA
Nonparametric Sensitivity Analysis for the Survivor Average Causal Effect: Sharp Bounds for Vaccine Efficacy
In studies in which the endpoint of interest is measurable only on a subset of units who experience a prior event, quantifying the …
Tue, May 24, 2022 10:00 AM
Berkeley, CA, USA
Efficient Estimation of Modified Treatment Policy Effects Based on the Generalized Propensity Score
Continuous treatment variables have posed a significant challenge for causal inference, both in the formulation and identification of …
Wed, Nov 17, 2021 10:30 AM
New York, New York, United States (remote due to COVID-19)
A Framework for Causal Segmentation Analysis with Machine Learning in Large-Scale Digital Experiments
We present an end-to-end methodological framework for causal segment discovery that aims to uncover differential impacts of treatments …
Fri, Nov 5, 2021 2:30 PM
Boston, Massachusetts, United States (remote due to COVID-19)
Nonparametric Estimation of the Generalized Propensity Score Based on the Highly Adaptive Lasso
Continuous treatment variables have posed a significant challenge for causal inference, both in the formulation and identification of …
Wed, May 19, 2021 6:30 AM
Oslo, Norway (remote due to COVID-19)